Real-World Applications of Bayesian Belief Networks
Bayesian networks are basically a subbranch of probability graphs. It basically is a graphical model on the basis of which one can represent variables or a set of variables and their dependencies on different conditions. For the representations, the Bayesian network used acyclic graphs. An Acyclic graph is a representation of a graph, in this kind of graph, there is no graphical cycle. There are two main types of acyclic graphs one is connected known as a tree and the other is disconnected which is known as a forest or collection of trees. There is another definition of a Bayesian graph presented in the article, the author explained that it is a kind of graphical model which represent the knowledge about something which is uncertain, every ode has some random value, and conditional probability can be observed on each edge of the graph. These conditional probability-based edges represent every knowledge of the random variable of the nodes.
Working of Bayesian Belief Network
Bayesian networks’ working is simple in nature. There are no complex variables or algorithms involved in the working of Bayesian belief networking unlike other machine learning or artificial intelligence models. These are simple graphical models having different edges and nodes. They have random variables available for working in the model, both dependent and independent relationships can be found between the variable using this technique. They can make models able to learn from the given data, they can become so strong after training and learning from the data that they can estimate the possibilities of some events. There are two main important parts to Bayesian belief networks, one is nodes which are basically the random variable in the tree or the data and the other one is the edge which represents the relationship between these nodes.
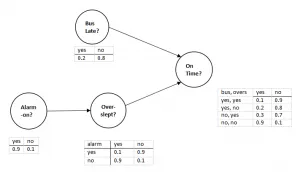
A Probabilistic Graphical Model of Bayesian Network
Applications of Bayesian Belief Network
Bayesian belief networks, nowadays are used in almost every field of machine learning, and artificial intelligence due to their less complex durability, and better approximation. This model is mostly used in those areas when a model is uncertain about the values of some event that has occurred at a specific area or a specific time. This helps the model to work in a competitive environment where the decision-making is on its own. From a technical point of view, there are different applications of Bayesian belief networks, some of the artificial intelligence, and machine learning fields that may have used these techniques are as; Image processing, CNN, GRN, Semantic Searches, Information Retrieval, and Medicine . A brief introduction of these fields in our daily lives is discussed as follows.
- GRN or Gene Regulatory Network . It is basically a field of biomedical linked with machine learning. The main focus of this field is DNA segmentation in a cell. In this technique, the DNA also comes closer to other substances in the cell. The DNA basically interact with them indirectly, here the word indirectly means it interacts through their interaction product. With the help of GRN, one can obtain the model behavior of the system using mathematical modeling. With the help of these mathematical modeling different predictions can be made and different weights can be assigned to them.
- There is also a large impact of Bayesian networking in the field of medicine as well. With the help of medicine, we have catered to many diseases since the stone age, the working of medicine is a prime application of Bayesian networking as it has some random probability about the health of the person.
- Biomonitoring is a field of biomedical instrumentation, in which the concentration of chemicals and their effects on the health of the person both short term and long term can be predicted. This can be explained by a simple example like there is an app that on the basis of a person’s EMI tells him about the number of calories he should have burned in order to stay active and healthy. When a person provides all the information to the app the application then keeps a check on the different parameters of the human body and warns the user of upcoming threats. All of these are purely based on probability and predictions.
- Document Classification is also an important application of the Bayesian networking system. In this technique, one can provide all the data to the system and the system on the basis of graphical probability tells us about the designated place for each book in the real-time library as keeping each book on the designated corner requires a lot of time and concentration.
- Information Retrieval is basically a technique that is used mostly by interrogation teams, and law enforcement agencies. On the basis of this one can extract useful information from CCTV footage or an interrogation.
- Semantic Search is basically improving the search result for a designated user. If a person watches Netflix more often than on his every search he has to find the ads related to different TV shows and movies.
- Image Processing has also used this technique sometimes in order to extract useful information from the image by improving the pixel and making the color. With the help of Bayesian networking, the model can be so well trained that it can increase the pixel where necessary and even decrease the coloring where there is no need. With the help of this one can obtain useful information from low-quality images as well.
- There is another technique named Spam filter. This technique is available in almost every mobile. The ML model in our mobile or cellular network helps to trace the unusual number and keep the message in the spam folder as it may be harmful to us or our data.
- Several more real-life applications of the Bayesian belief networks include turbo code, system biology, tumor detection, and safe city projects.
Learn how Algoscale can help, here !